June 3, 2024
Article
3 Changes Genomics Microscopes Bring to Understanding Disease
View all topics
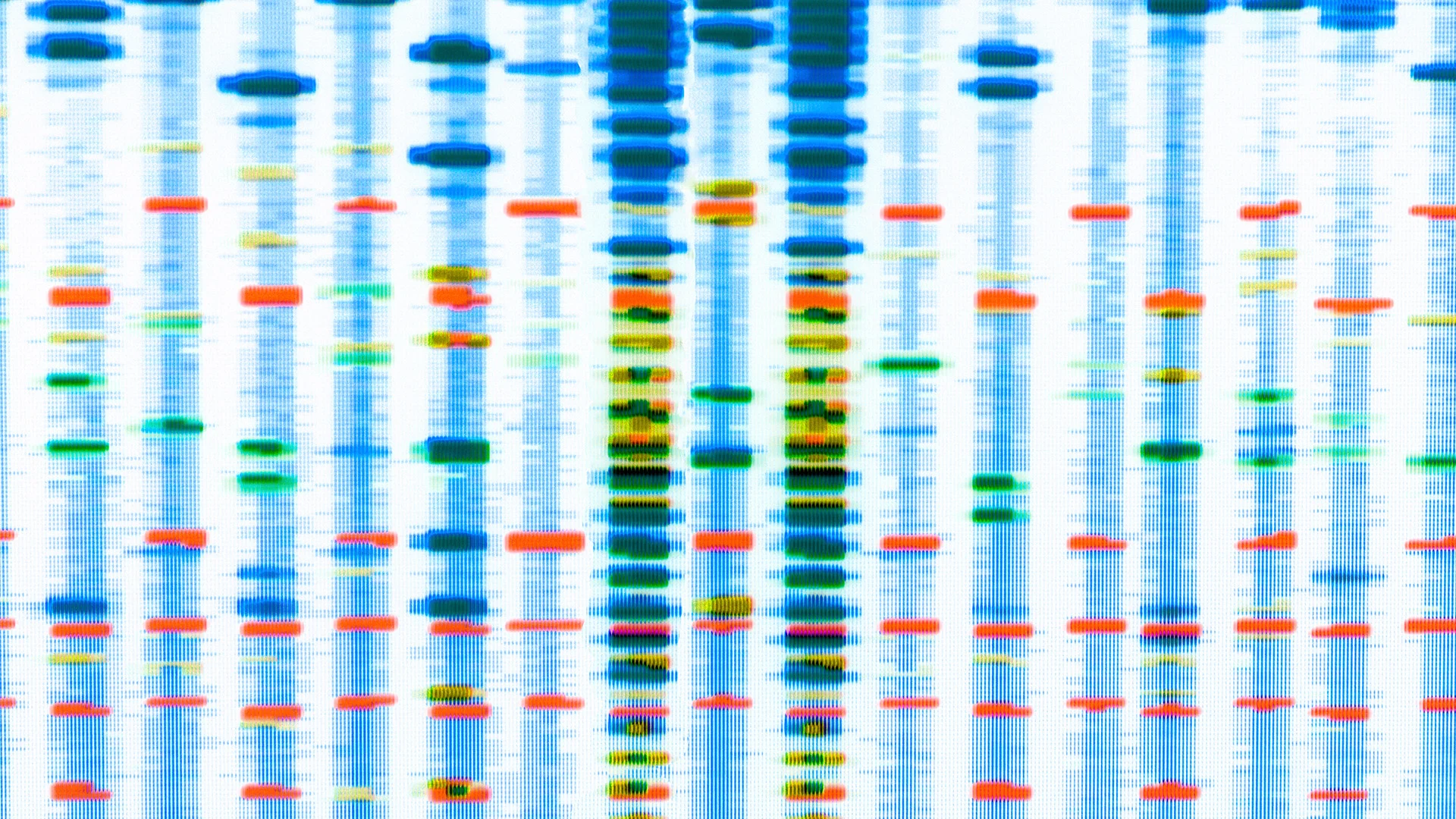
Overview
Trailblazing computational biologist Manolis Kellis, PhD, whose MIT laboratory works at the interface of computer science and biology, likens his work interface to a "genomics microscope."
New approach enables researchers to observe millions of cells at single-cell resolution,
The causes of common diseases such as Alzheimer’s and cancer, as well as thousands of rare diseases, are coming into sharper focus thanks to the convergence of the genomics revolution, the plummeting cost of sequencing — and AI.
“We’re in for a dramatic transformation,” said trailblazing computational biologist Manolis Kellis, PhD, whose MIT laboratory works at the interface of computer science and biology, which he likened to working with a ‘genomics microscope.’
This highly interdisciplinary approach enables researchers to observe millions of cells at single-cell resolution, measure nucleotides of every cell in the body, understand mutations, and recognize how the cells take their own identity through epigenomics.
Kellis’ lab is developing methods discovery, characterization and validation of genomics not just of single cells, but also for models of diseases ranging from Alzheimer’s, schizophrenia and other neuroscience illnesses, as well as of metabolism, obesity and diabetes and of cancers, among other research areas.
Based on his investigations, Kellis told Cure he believes adding AI to genomics puts medicine at the threshold of three paradigm shifts in understanding and curing disease:
Paradigm Shift Number 1: Hypothesis Follows the Data
“It used to be that you would come up with a hypothesis and gather a bunch of data —experiments followed the thinking. The first paradigm shift is massive data, and then the hypothesis follows the data,” explained Kellis.
In the past, in many examples with human genetics, “we thought that a gene was causally implicated in a disease because the three people who had the disease also had a mutation. Then we sequenced everyone. We found that there are hundreds of other people that have the mutation. It turns out it's not causal.”
So, by having massive data as a resource for everyone, investigators now can have much more unbiased hypotheses. “We can truly go where the light is taking us,” he said.
Paradigm Shift Number 2: Genetics Provides Causality
The second paradigm shift is in pinning down the causes of disease. Epidemiology, Kellis explained, has been about correlation. For example, showing that people who drink Starbucks three times a week have better health outcomes. Is it because expensive coffee gives better health outcomes or is it because people with better socioeconomic status have better healthcare and can afford a $6 coffee?
But causation, rather than correlation, can provide more clarity about diseases, and “causality comes from genetics,” said Kellis. Many beautiful papers show drugs that have genetic support are two times more likely to succeed or more than drugs without genetic support.
“Being able to show that there's a mutation that correlates with the disease outcome means that if I intervene in that same spot, I will get to the disease outcome. Now we know where to intervene.”
Paradigm Shift Number 3: From Single Questions to Foundation Models
With genomics, proteomics, transcriptomics, epigenomics, and more, data on the molecular basis of disease has never been more plentiful. With this data, AI foundation models are transforming the study of disease to understand the basic functioning of the cell.
“We can start asking AI, what drug should I develop? How should I change the function of a protein to move more in that space, in a gradient of how all cells relate to each other in terms of the expression of all their genes?” said Kellis. “On the pharmaceutical side, we can start with genetics for causality and knowing where to intervene. But then when we have the circuitry, we find the knobs that we should be turning. And with high-throughput biology, we can screen drugs and see which ones are shifting the transcriptional space in the direction away from disease.”